Title: All-Optical Data Processing with Photon Avalanching Nanocrystalline Photonic Synapse
Authors: A. Bednarkiewicz, M Szalkowski, M Majak, Z Korczak, M Misiak, S Maćkowski
Journal: Advanced Materials
DOI: 10.1002/adma.202304390
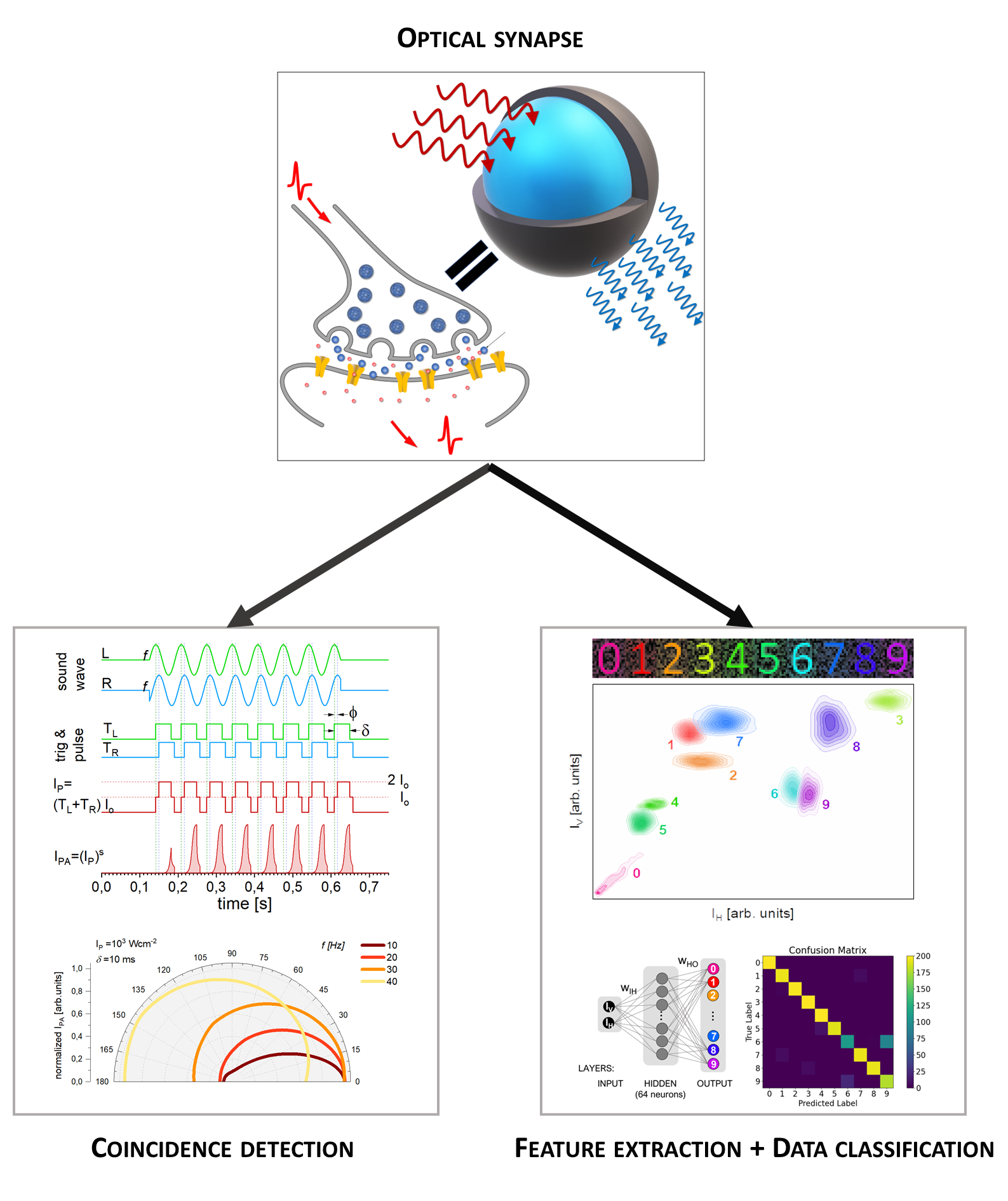
In recent years, computing needs have begun to surpass the capabilities of the modern von Neumann architecture. As a result, alternative computing approaches are being explored. One of the interesting approaches is reservoir computing, which emulates functionalities of neurons and synapses, including parallel operations, associativity, positive paired pulse facilitation (PPF), and the all-or-nothing law. These characteristics hold promising potential for more efficient computing capabilities in terms of power consumption and time usage.
In a recent article All-Optical Data Processing with Photon Avalanching Nanocrystalline Photonic Synapse a new inorganic material based on photon avalanche emission has been introduced, with the potential for use in reservoir computing. The 9800 nm emission from 8%Tm3+ doped nanoparticles under 1059 nm excitation have been utilized. These synapses are mimicking the operating principles of biological synapses, as their optical response varies based on the modulation of spatio-temporal optical excitation signals. The optical synapse demonstrates short-term memory and significantly enhanced paired pulse facilitation (PPF) compared to other previously reported materials. These effects have been demonstrated by both theoretical analysis and experimental results and originate from the long-living state and highly nonlinear power-dependent luminescence emission characteristic of lanthanide photon avalanche materials. The optical synapse's short-term memory behavior resembles that of the animal Nucleus Magnocellularis (NM), showcasing the ability to detect coincidences between two phase-shifted optical pulses. Additionally, optical synapse exhibits feature extraction capabilities from noisy digital images. Such feature extraction allowed for the compression of a 16x19 pixel matrix into a two-element vector. The vectors extracted from each digit were subsequently utilized as inputs for a simple, conventional one-layer neural network (NN). Based on these compressed data, the NN achieved a 93% accuracy rate in classifying digits.